How Can Data Visualization Help in the Banking and Finance Sector?
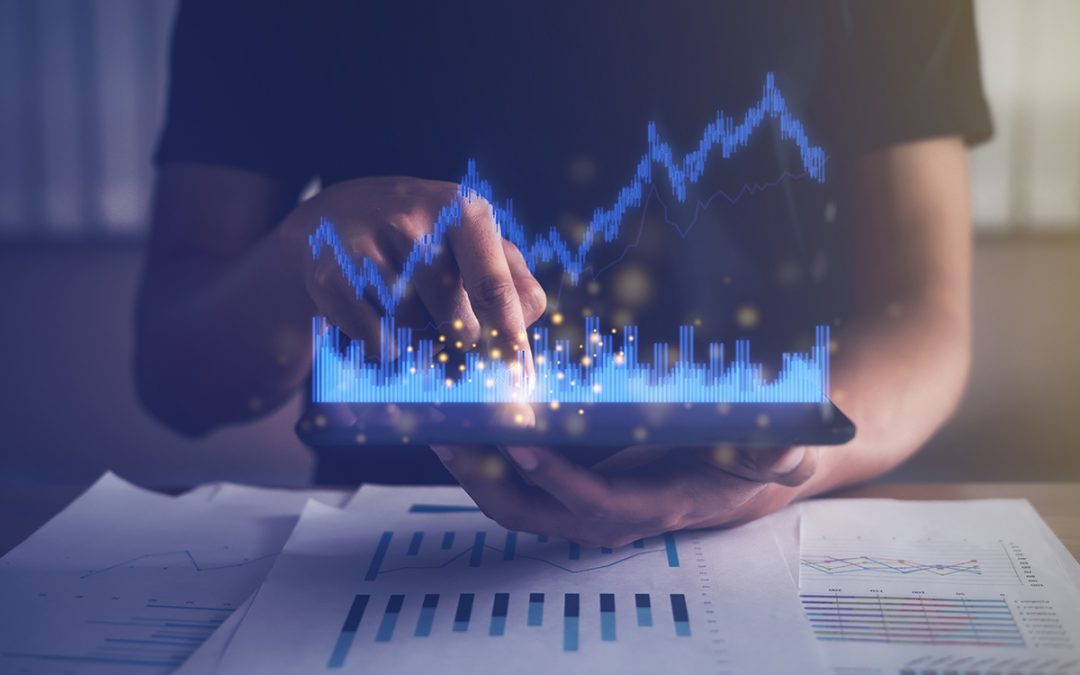
Real insights come from looking at the world through data and then figuring out how it relates to real insights by talking to customers. Analytics is becoming a competitive necessity for businesses, whether financial services, consumer goods, travel, transportation, or industrial products.
Across all industries, companies that are more analytically driven see three times as much financial growth as their competitors, who are not as analytical. Pharmaceuticals and medical products, insurance, energy, materials, and agriculture are some industries with the most advanced analytics.
But banking, which has been using data for a long time, starts from the best place.
The rising value of insights based on data
In today’s fast-paced business environment, it’s important for finance teams and banking institutions to find data-driven insights and communicate them well. Understanding numbers is still a valuable skill, but it’s also becoming more important to share what the nuances in data mean and why they can be crucial.
From nice-to-have to must-have: Data visualization in Banking Services.
Today business performance is turned into insights with the help of automated comments available in data visualizations.
Data visualization equips a command center with a customized alert system.
Data Visualization directs executive attention to the most important areas based on the insights gained. It allows to drill down into critical KPIs and corresponding key focus areas thus identified.
Furthermore, inbuilt tagging in data visualization assists teams in workflow assignments.
Present-Day Challenges in Data Visualization.
Data visualization is compelling when used correctly because it shows a clear turning point and can make a much stronger case than words or simple data charts. But more often than not, analysts spend about 80% of their time loading data and getting it ready and only 20% of their time making analytics.
This means more time is spent cleaning, reforming, and putting together messy, unrelated data than visualizing and analyzing the results. So, the key is automating as much of the data load as possible to make it easier.
How can banks benefit from Data Visualization?
Analytics is a strategic theme for banks, but most have trouble connecting their high-level analytics strategy to a targeted selection and prioritization of use cases and putting them into action in an organized way. Banks use data visualization in commercial, risk, innovation, and technology areas. It helps align the priorities of analytics with the strategic vision.
Integrating analytics into decision-making and enhancing execution.
One mandate for data visualization is to build advanced analytics assets and teams so businesses can grow. Most banks have been able to start single, stand-alone projects in advanced analytics that work well, but few have turned them into large-scale, efficient operations. Broader use of visualization reveals transformative opportunities and makes it possible to connect with third-party vendors, which allows competence development.
Investing in crucial analytics roles.
Banks are hiring more data engineers, data scientists, visualization specialists, and machine-learning engineers to meet the growing demand for people with these technical skills. With the growing importance of data visualization, the need for translators is also increasing. Translators are a vital link between business and analytics. They help data scientists understand business problems and priorities and ensure analytics insights are shared with business units.
Allowing the user revolution to happen.
Banks have a lot of great data sources that can be used in many different ways, but their data practices tend to be narrow and focused on regulations. So, as data visualization practices permeate, high-quality data is more readily usable to build analytics use cases.
Conclusion
As competition in the financial services industry gets tougher, banks must take a data-driven approach to stay in the game. One important thing to remember when using data visualizations in reports and presentations is that too much detail, no matter how it’s presented, makes it hard for people to understand the main points.
It’s important to remember that these reports and presentations are meant to send clear messages. So, instead of putting a bunch of different graphs in a report, finding the one that best conveys the message and then explaining what it means will be much more effective.
Even if more details are needed, less is often more.
View