A Guide on the Regulatory Changes Impacting Banking Transformation
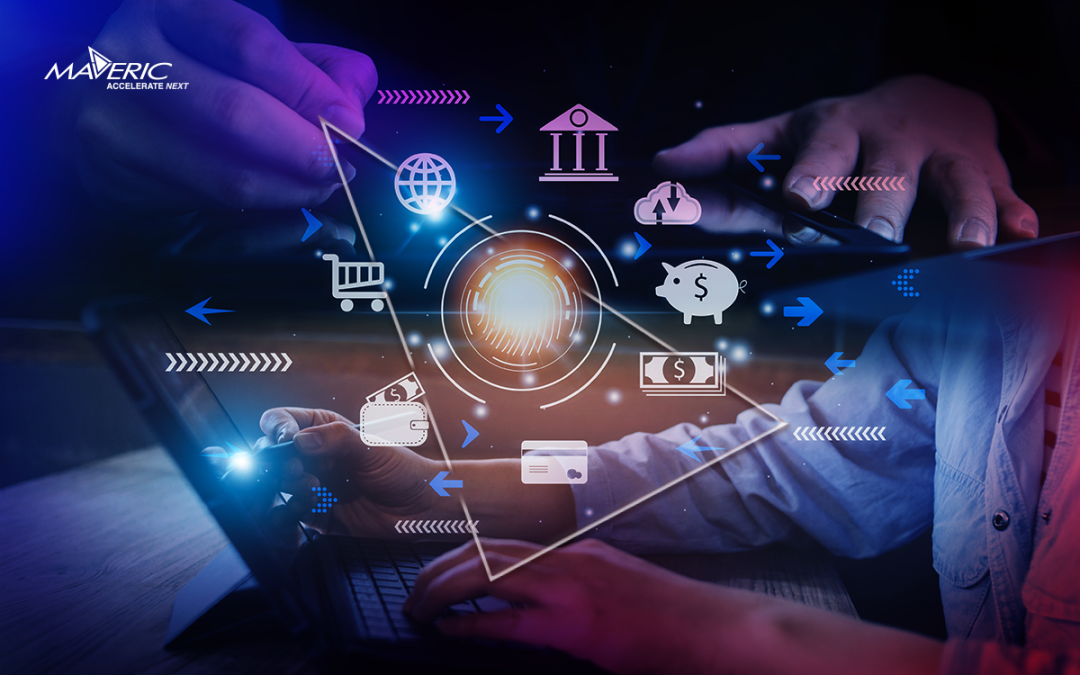
In the fast-paced banking realm, where technology and customer expectations constantly evolve, regulatory changes are a cornerstone shaping the trajectory of Banking Transformation. As financial institutions navigate the intricate regulatory compliance landscape, the symbiotic relationship between adhering to regulations and undergoing transformative change becomes increasingly apparent.
In the wake of rapid technological advancements, banking regulations have become more critical than ever. The increasing complexity of financial transactions, coupled with the growing threats in the digital space, necessitates a robust regulatory framework. Here’s why the role of banking regulations is crucial in Today’s world:
1. Adapting to Technological Evolution:
Banking regulations ensure that financial institutions keep pace with technological evolution. From adopting cloud computing to leveraging artificial intelligence, banks must adhere to regulatory standards to integrate these technologies securely.
2. Mitigating Financial Risks:
The global interconnectedness of financial markets requires comprehensive regulations to mitigate risks. Regulatory changes are designed to address emerging risks and ensure the financial system’s stability amid economic uncertainties.
3. Protecting Consumer Interests:
With an increasing reliance on digital transactions, regulatory changes aim to protect consumer interests by enforcing data privacy and security measures. This is particularly crucial in an era where data breaches and identity theft pose significant threats.
4. Promoting Financial Inclusion:
Banking regulations also play a role in promoting financial inclusion. By setting standards for digital banking and payment services, regulators contribute to making financial services accessible to a broader population.
The Symbiosis of Regulatory Compliance and Banking Transformation
1. Digital Imperative and Regulatory Compliance Services:
Banks are compelled to digitize operations, driven by regulatory bodies’ demands for transparency and efficiency. According to a recent survey, 85% of banks have increased their investment in regulatory compliance services over the last two years.
2. Regulatory Reporting Requirements:
Stringent reporting requirements necessitate advanced data management systems, fueling the adoption of next-gen technologies. A Leading Bank implemented a cloud-based solution to meet intricate regulatory reporting demands, reducing reporting errors by 30%.
3. RegTech Solutions for Growth:
Banks leveraging Regulatory Technology (RegTech) solutions streamline compliance processes, unlocking resources for innovation. A noteworthy FI witnessed a 25% reduction in compliance costs after integrating AI-driven RegTech for regulatory reporting.
Impact on Banking Customers
These changes translate into a more secure, transparent, and seamless experience for a banking customer. Enhanced regulatory compliance ensures the safety of customer data and transactions. Moreover, as banks optimize their operations to meet regulatory standards, customers benefit from improved services, quicker transactions, and innovative financial products.
Noteworthy Examples
1. JP Morgan Chase:
JP Morgan Chase invested $10 million in a comprehensive RegTech platform, streamlining its compliance processes. The move resulted in a 20% reduction in regulatory reporting time.
2. HSBC:
HSBC adopted advanced analytics for regulatory compliance, leading to a 15% increase in efficiency. The bank redirected saved resources towards customer-centric digital initiatives.
3. Bank of America:
Bank of America embraced blockchain for regulatory reporting, reducing instances of errors and ensuring real-time compliance. This step enhanced the bank’s reputation for reliability.
The Crucial Nature of Regulatory Changes in Banking Transformation
1. Ensuring Compliance and Stability:
Regulatory changes are a compass guiding banks toward compliance, providing stability and trust in the financial ecosystem. The stringent requirements set by regulatory bodies are designed to safeguard the interests of financial institutions and their customers.
2. Facilitating Technological Advancements:
As technology becomes an integral part of banking operations, regulatory changes often drive the adoption of advanced technologies. Compliance requirements push banks to invest in next-gen solutions, leading to improved efficiency, transparency, and security.
3) Fostering Innovation:
While compliance is non-negotiable, regulatory changes catalyze innovation. Banks must rethink traditional processes and develop innovative products and services that meet regulatory standards and customer expectations.
4) Protecting Customers and Data:
The evolving regulatory landscape is designed to address the challenges the digital age poses. With cyber threats rising, regulatory changes focus on enhancing data security and protecting customers from potential risks, contributing to a safer banking environment.
5) Enhancing Customer Experience:
Contrary to the perception that regulatory changes might hinder customer experience, they can improve its enhancement. By streamlining processes, reducing risks, and ensuring compliance, banks can provide a more seamless and trustworthy customer experience.
Conclusion
The interplay between regulatory changes and banking transformation is a defining factor in the industry’s evolution. Banks that successfully navigate this landscape stand to gain compliance, foster innovation, and deliver enhanced customer value. Staying abreast of changes and strategically embracing transformative technologies will be pivotal for sustained success as the regulatory journey unfolds.
About Maveric Systems
Starting in 2000, Maveric Systems is a niche, domain-led Banking Tech specialist partnering with global banks to solve business challenges through emerging technology. 3000+ tech experts use proven frameworks to empower our customers to navigate a rapidly changing environment, enabling sharper definitions of their goals and measures to achieve them.
Across retail, corporate, and wealth management, Maveric accelerates digital transformation through native banking domain expertise, a customer-intimacy-led delivery model, and a vibrant leadership supported by a culture of ownership.
With centers of excellence for Data, Digital, Core Banking, and Quality Engineering, Maveric teams work in 15 countries with regional delivery capabilities in Bangalore, Chennai, Dubai, London, Poland, Riyadh, and Singapore.
View