Quick-Win AI Strategies for Banks
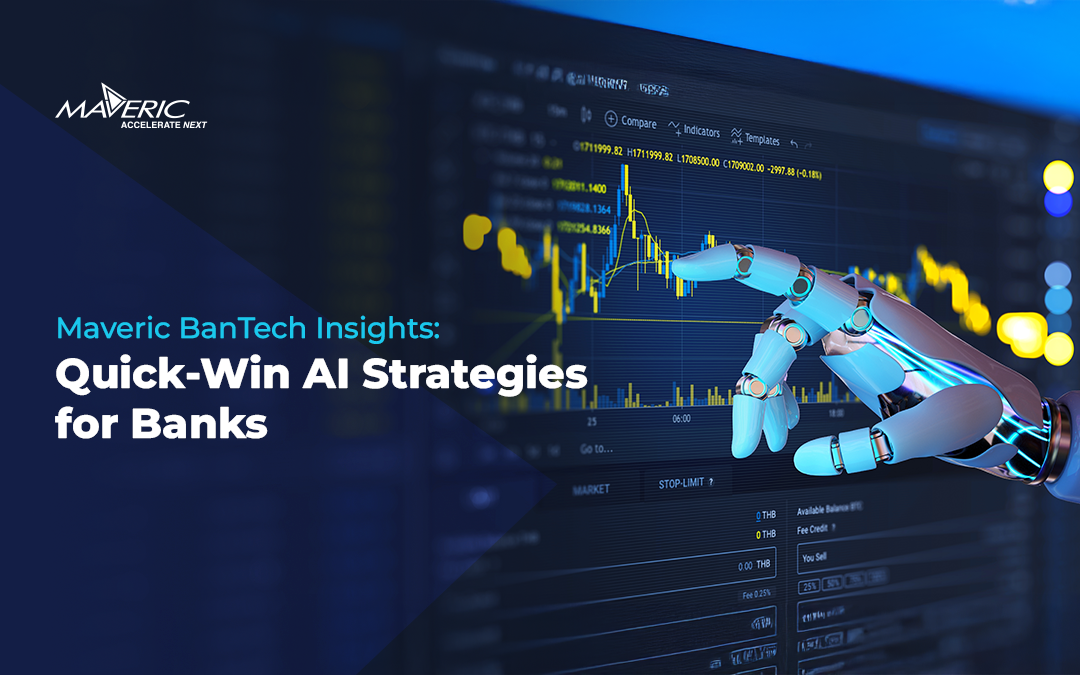
Spotlight on AI in Banking
Key Principles and Considerations
Banks have been exploring AI applications for over a decade, with Natural Language Processing (NLP) and Machine Learning (ML) being at the forefront. These technologies have been primarily focused on two areas:
1. NLP Applications:
These have revolved around converting voice to text, processing text in multiple formats, and converting text back into voice in certain applications.
2. ML Applications:
The focus here has been on pattern recognition, identifying trends, and making forecasts.
As banks continue their AI journeys, they are faced with several choices. One of the first considerations is the potential for incorporating unstructured data. Can their current platform handle this type of data, or does it require an upgrade?
Additional questions include whether integrating new features (like switching from a traditional lexicon search to a semantic search, or transitioning from ML to Deep Learning) would enhance efficiency. Moreover, banks must consider the role of GenAI in reducing manual intervention and whether it can integrate with their existing platforms. This also leads to the question of whether upgrading their current tech stack or switching to a newer stack could yield improved performance and operational efficiency.
Another critical point is sustainability. Can the current AI infrastructure be managed by the business teams with minimal technical expertise, or is it heavily dependent on specialized resources?
Market Insights: The Current State of AI in Banking
Financial institutions that have ventured into AI typically face the following scenarios:
1. Custom Platform Development:
Banks often build custom platforms using a mix of open-source and commercial tools.
2. Training and Fine-Tuning:
These platforms are trained using the bank’s own data to achieve production-grade accuracy.
3. NLP with Structured Data:
Banks have predominantly used structured data for their NLP-based searches.
4. Periodic Recalibration of ML Models:
To keep their models up to date, banks often perform regular recalibrations based on application requirements.
Despite these efforts, the gap between hype and implementation remains wide. While large banks and tech companies have made strides, many smaller institutions continue to struggle with scaling AI solutions.
Impact of GenAI
GenAI is projected to provide a significant boost to the banking sector. McKinsey’s Global Institute forecasts GenAI could add $200-$340 billion annually to the global banking sector by increasing productivity. A 2024 Bain & Company survey found that U.S. financial firms saw an average productivity gain of 20% with GenAI applications. However, the challenge lies in leveraging this potential across banks of varying sizes and technological capabilities.
Industry Best Practices
Strategies to Leverage Existing AI Capabilities
Banks are at a crossroads when it comes to AI adoption. Rather than pursuing wholesale overhauls of their technology stacks, many banks can achieve rapid success by extending their existing AI capabilities. This involves considering the following strategies:
1. Integrate New-Age Tools and Platforms:
Banks can enhance their current platforms by integrating newer AI tools and platforms that extend the functionality of existing solutions. This may include integrating GenAI into customer service, risk assessment, and fraud detection systems.
2. Component-Level Transformations:
Instead of overhauling entire systems, banks could make selective, incremental changes to their infrastructure. For example, upgrading certain parts of their platform to support unstructured data or transitioning from traditional ML models to Deep Learning models could deliver quick wins.
3. Upgrade the Entire Tech Stack:
For institutions with legacy infrastructure that limits their AI potential, a more radical approach of migrating to a newer, more advanced technology stack may be necessary. This can involve switching to cloud-based, AI-optimized platforms or leveraging SaaS solutions to speed up implementation.
Cross-Industry Learning
Banks can learn from industries that have successfully adopted AI, such as retail and e-commerce, which have pioneered AI-driven customer experience platforms, chatbots, and personalization algorithms. By adopting best practices from these industries, banks can accelerate their own AI adoption in areas such as fraud detection, customer service, and regulatory compliance.
Future Trends: What's Next for AI in Banking?
The future of AI in banking is likely to evolve along two major paths:
1. Transition to Deep Learning (DL) and Agentic AI:
As AI tools mature, there will be a shift toward deep learning models, which can process large amounts of unstructured data (such as text, audio, and images) and make more sophisticated predictions. Agentic AI, which can autonomously make decisions and take actions based on learned patterns, will also become more prevalent. This could lead to more personalized banking experiences and advanced fraud detection systems.
2. AI-Driven Regulatory Compliance:
With increasing regulation in the financial industry, AI is likely to play a larger role in compliance. AI tools that can automatically monitor and adapt to changes in regulatory requirements will help banks stay ahead of the curve. This includes using NLP to analyze new regulations and ML models to ensure compliance across operations.
3. Collaboration Between Financial Institutions and Tech Companies:
As AI becomes more complex, banks will need to partner with technology providers to gain access to the latest innovations. These collaborations will be crucial in accelerating AI adoption and driving industry-wide digital transformation.
Conclusion
The case for AI in banking is undeniable, and the potential benefits are immense. However, to unlock these benefits, banks must carefully assess their current capabilities and avoid attempting large-scale, disruptive changes. Instead, enhancing and extending existing AI platforms can lead to significant improvements in productivity and customer experience. By focusing on incremental upgrades, adopting best practices from other industries, and ensuring regulatory compliance, banks can position themselves for success in the evolving AI landscape.
We hope this series serves as a valuable reference for banking leaders as they navigate their AI journey. We welcome your perspectives and experiences on how AI is transforming the industry.
With 25 years of expertise in BankTech, Maveric has been at the forefront of driving transformative solutions in the banking domain.
Through the Maveric BankTech Insights newsletter, we bring you deep insights overcome digital friction in Banking that not only attract but also retain and delight customers for the long term. Wish to know more? Subscribe to our Newsletter